By Judea Pearl & Dana Mackenzie (2018)
Pages: 432, Final verdict: Great-read
I had countless discussions with my thesis supervisor on the causes of road traffic accidents. I was proposing to build an AI model to predict which variables had more impact and all I had was an enormous dataset. For a student, starting to create an hypothesis to explain the influence of the causal variables in the outcomes seemed like running in circles. A few months later I found what could have helped me to run in a straight line - The Book of Why - and its promise to establish the causal revolution.
The book couldn’t have a better author. It is written by one of the causal inference founders, Judea Pearl. I thought this was the breakthrough moment for statistical science, causal problems’ modelling and for the ordinary people that wished to understand it. In a labyrinth of incoherences and intricate explanations, Judea is still able to shape the field of cause and effect. He creates the Ladder of Causation by identifying vocabulary differences between how causal questions and scientific theories are communicated, a gap that harms the understanding of causal inference.
“If we want our computer to understand causation, we have to teach it how to break rules. We have to teach it the difference between merely observing an event and making it happen.” - Judea Pearl
Climbing the Ladder of Causation
The Ladder of Causation represents the three levels of causation - Association, Intervention, and Counterfactuals - and a classification for cognitive ability:
- Association - Focus on observation and relates to questions such as “What if I see…?” and “How are the variables related?”.
- Intervention - Stands for data manipulation on controlled tests, originating questions such as “What if I do…? How?”, “What would Y be if I do X?” and “How can I make X happen?”.
- Counterfactuals - Judea’s major thesis to create predictions using imagination and bringing a new perspective to causation. His focus is on questions such as “What if I had done…? Why?”, “What if X had not occured?” and “What if I acted differently?”.
Judea Pearl shapes the world of cause and effect as a world of systems where each outcome is formed by a set of causal variables that move across time. He puts on notice the existence of a single root cause and passive observations of data. There’s a reason why, as kids, we’ve listened to our parents saying we should listen to the elderly - they have seen much already. Judea’s vision gives perspective to shape hypotheses just like the elderly have perspective over things we don’t know about. It works as a stimulus to search for the set of whys, the causation, and to break with the past.
“We begin learning causes and effects before we understand language and before we know any mathematics. (...) It is because of this robustness, I conjecture, that human intuition is organized around causal, not statistical, relations.” - Judea Pearl
The chapters are chronologically ordered to follow the history of causal inference. However, it becomes frustrating as it leads to a hunt of past failures on dealing with causation before the book’s theory is explained. As the solution for the failures of the past, Judea Pearl presents his theory - the causal diagrams.
It relies on connecting the causal variables using arrows. Each arrow represents a causal relation which strength is represented by a coefficient. Judea’s claim hangs on the need of creating a diagram to state assumptions and relations beforehand. He pictures an hypothesis supported on causation first and uses data to prove the diagram’s claim after. It contrasts with correlation as the approach is not exclusively based on observation nor on tests with manipulated data in a single instance in time. This is Judea’s innovative assertion to approach an hypothesis. But how he frames its application is a problem.
Unfortunately, he only supports such claims using already solved problems of the past like the smoking impact on lung cancer (see diagram below). Some of the examples are backed by exhausting equations and hazy assumptions, inadequately explaining how it applies to today’s world and beyond statistical science.The book partially misses the area where I saw the most potential.
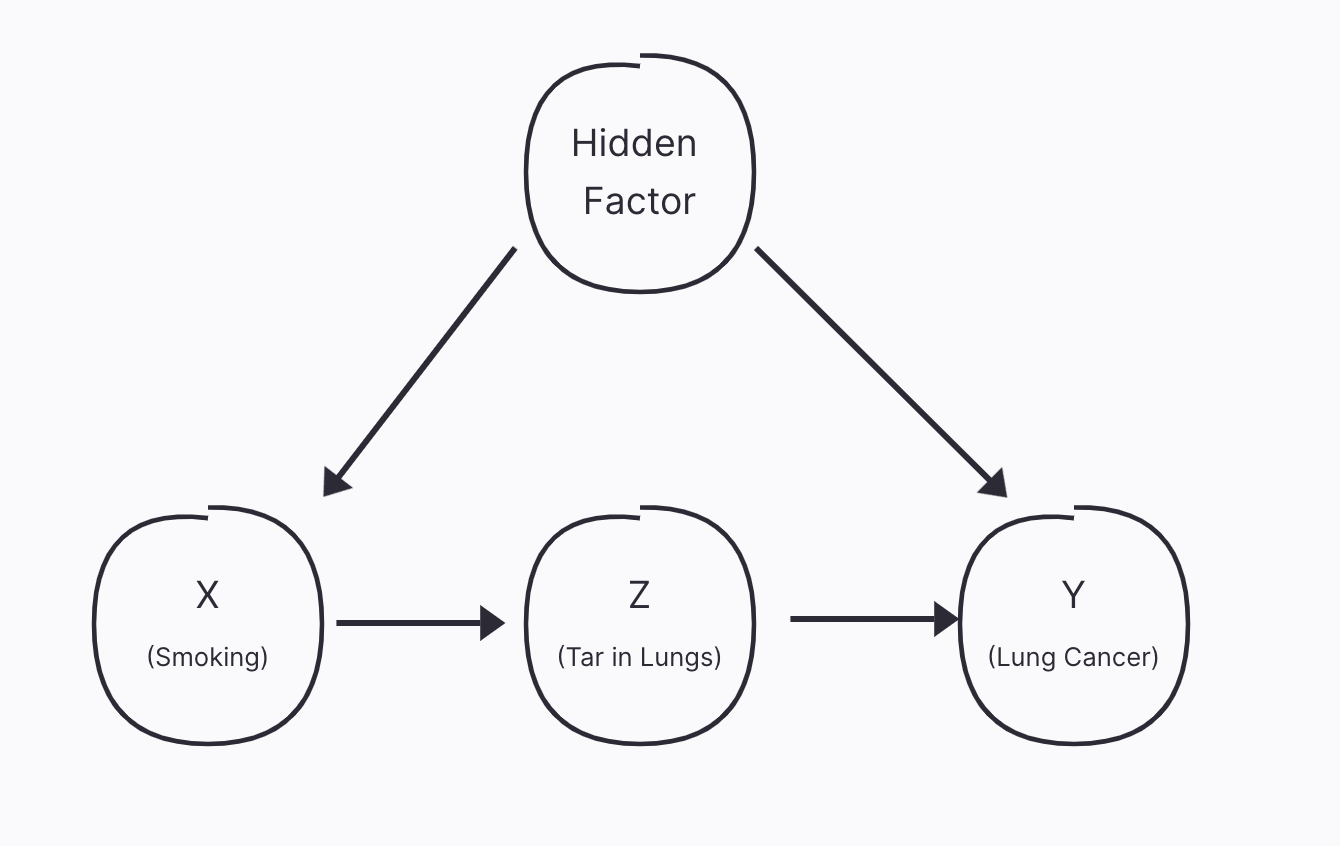
By projecting causation principles in today's activities, you can see how causation is underrated. In product development, the need a product aims to address exists due to a set of causal variables that ultimately affect the consumer. Those variables can be mapped to find the problem’s origin, the set of causes that leads the consumer to the product. It also changes the sales mindset. Bob Moesta, President & CEO at Re-Wired Group, explained in an interview a couple years ago why there’s a misconception on buying habits due to marketers incorrect usage of correlation and causation. In his words, it’s not because you are 52, live in a particular zip code, and have this kind of car that you will use a product. There is always a set of causes underneath.
Bottom Line
I can now highlight a significant reason for my early struggles in the thesis. I fell into the temptation to use correlation because all I had was the dataset and no previous experience on causation. I crammed over several thousands of instances, searching for patterns that would allow me to create an hypothesis. But, as mentioned by Judea in the last part of the book, that’s exactly what AI models are supposed to do. They act on the first rung only. But not humans. The answer I needed was two rungs above on the Ladder of Causation and we can climb it.
If you deal with data regularly or if you feel trapped in data observation, this is a good book to gain perspective and develop a wider understanding on why to use the data. But you should be prepared to embrace the technical explanations and mathematical references from Judea - if that is not what you wish, there’s probably simpler correlation and causation references out there too.
Further learning:
- Buy the book
- Bob Muesla interview in Shaping Chaos podcast
- Amplitude blog post about causation and correlation differences in Product Development
- Khan Academy - Correlation & Causation
- Causation, Prediction, and Search
This article was written by a guest author, Bruno Teixeira.